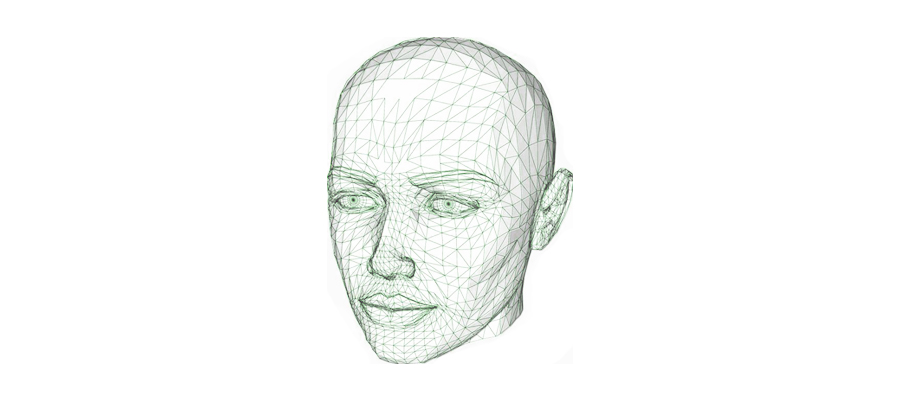
在相机应用中,实时贴纸、实时瘦脸是比较常见的功能,它们的实现基础是人脸关键点检测。本文主要介绍,如何在 GPUImage 中检测人脸关键点。
前言
我们要通过某一种方式,获取视频中每一帧的人脸关键点,然后通过 OpenGL ES 将关键点绘制到屏幕上。最终呈现效果如下:
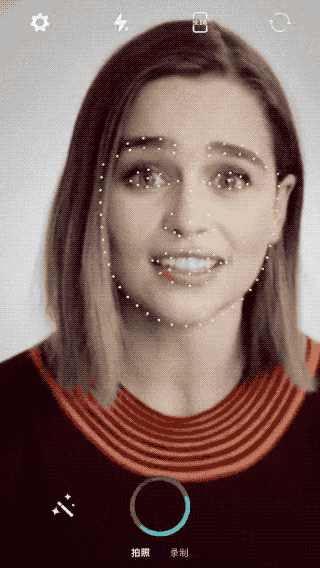
这里分为两个步骤:关键点获取、关键点绘制。
一、关键点获取
在苹果自带的 SDK 中,已经包含了一部分的人脸识别功能。比如在 CoreImage、AVFoundation 中,就提供了相关的接口。但是,它们提供的接口功能有限,并不具备人脸关键点检测功能。
我们要在视频中进行实时的人脸关键点检测,还需要借助第三方的库。这里主要介绍两种方式:
- Face++
- OpenCV + Stasm
1、Face++
1、简介
Face++ 的人脸关键点 SDK 是收费的,但是它也提供免费试用的版本。
在免费试用的版本中,试用的 API Key 每天可以发起 5 次联网授权,每次授权的时长为 24 小时。也就是说,在不删除 APP 的情况下,只要测试设备不超过 5 台,就可以一直使用下去。
这对于开发者来说还是非常友好的,而且 Face++ 的注册集成也比较简单,建议大家都尝试一下。
2、如何集成
人脸关键点 SDK 的集成可以参照 官方文档 ,先注册再下载 SDK 压缩包,压缩包里有详细的集成步骤。
3、如何使用
人脸关键点 SDK 的使用主要分为三步:
第一步:发起联网授权
授权的操作不一定发起网络请求,而是会先检查本地的授权信息是否过期,过期了才会发起网络请求。
1 2 3 4 5 6 7 8 9 10 11 12
| @weakify(self); [MGFaceLicenseHandle licenseForNetwokrFinish:^(bool License, NSDate *sdkDate) { @strongify(self); dispatch_async(dispatch_get_main_queue(), ^{ if (License) { [[UIApplication sharedApplication].keyWindow makeToast:@"Face++ 授权成功!"]; [self setupFacepp]; } else { [[UIApplication sharedApplication].keyWindow makeToast:@"Face++ 授权失败!"]; } }); }];
|
第二步:初始化人脸检测器
授权成功后,开始人脸检测器的初始化。初始化过程会进行模型数据加载,然后对识别模式、视频流格式、视频旋转角度等进行设置。
1 2 3 4 5 6 7 8 9
| NSString *modelPath = [[NSBundle mainBundle] pathForResource:KMGFACEMODELNAME ofType:@""]; NSData *modelData = [NSData dataWithContentsOfFile:modelPath]; self.markManager = [[MGFacepp alloc] initWithModel:modelData faceppSetting:^(MGFaceppConfig *config) { config.detectionMode = MGFppDetectionModeTrackingRobust; config.pixelFormatType = PixelFormatTypeNV21; config.orientation = 90; }];
|
第三步:检测视频帧
人脸检测器初始化成功后,可以对视频流每一帧进行检测,这里传入的是 CMSampleBufferRef
类型的数据。由于顶点坐标的范围是 -1 ~ 1
,所以还需要根据当前的视频尺寸比例,对识别的结果进行坐标转换。
1 2 3 4 5 6 7 8 9 10 11 12 13 14 15 16 17 18 19 20 21 22 23 24 25 26 27 28 29 30 31 32 33 34 35 36 37 38 39
| - (float *)detectInFaceppWithSampleBuffer:(CMSampleBufferRef)sampleBuffer facePointCount:(int *)facePointCount isMirror:(BOOL)isMirror { if (!self.markManager) { return nil; }
MGImageData *imageData = [[MGImageData alloc] initWithSampleBuffer:sampleBuffer]; [self.markManager beginDetectionFrame]; NSArray *faceArray = [self.markManager detectWithImageData:imageData]; NSInteger faceCount = [faceArray count]; int singleFaceLen = 2 * kFaceppPointCount; int len = singleFaceLen * (int)faceCount; float *landmarks = (float *)malloc(len * sizeof(float)); for (MGFaceInfo *faceInfo in faceArray) { NSInteger faceIndex = [faceArray indexOfObject:faceInfo]; [self.markManager GetGetLandmark:faceInfo isSmooth:YES pointsNumber:kFaceppPointCount]; [faceInfo.points enumerateObjectsUsingBlock:^(NSValue *value, NSUInteger idx, BOOL *stop) { float x = (value.CGPointValue.y - self.sampleBufferLeftOffset) / self.videoSize.width; x = (isMirror ? x : (1 - x)) * 2 - 1; float y = (value.CGPointValue.x - self.sampleBufferTopOffset) / self.videoSize.height * 2 - 1; landmarks[singleFaceLen * faceIndex + idx * 2] = x; landmarks[singleFaceLen * faceIndex + idx * 2 + 1] = y; }]; } [self.markManager endDetectionFrame];
if (faceArray.count) { *facePointCount = kFaceppPointCount * (int)faceCount; return landmarks; } else { free(landmarks); return nil; } }
|
2、OpenCV + Stasm
1、简介
OpenCV 是一个开源的跨平台计算机视觉库,实现了图像处理方面的很多通用算法。Stasm 是用于检测人脸特征的开源算法库,依赖于 OpenCV 。
我们知道,iPhone 屏幕的刷新频率可以达到 60 帧每秒。在相机预览时,出于功耗方面的考虑,一般会将帧率限制到 30 帧每秒左右,且不会引起明显的卡顿。
所以,我们要对每一帧数据进行识别,则要求每一帧的识别时间要小于 1 / 30 秒,否则图像数据的渲染操作就要等待识别结果,从而导致帧率下降,引起卡顿。
遗憾的是,采用 OpenCV + Stasm 的方式,每一帧的识别时间是超过 1 / 30 秒的。它或许更适合用来做静态图片的识别。
所以也更推荐使用 Face++ 的方式。
2、如何集成
OpenCV 通过 CocoPods 的方式来引入:
OpenCV2-contrib 相比于 OpenCV2 多包含了一些拓展包,比如 face 模块,而 Stasm 算法库需要依赖 face 模块。
Stasm 算法库可以从 这个地址 下载,需要将 stasm 和 haarcascades 文件夹都加入工程中。
3、如何使用
人脸关键点的识别主要通过调用 stasm_search_single
函数来实现。
由于这个方法的检测时间较长,因此我们在将视频帧数据传入之前,会先做单通道化、尺寸压缩等处理。这样的话, Stasm 拿到的每一帧的数据量会减少,可以有效地缩短检测的时长,但相应地也会损失检测的精度。
关键的代码:
1 2 3 4 5 6 7 8 9 10 11 12 13 14 15 16 17 18 19 20 21 22 23 24 25 26 27 28 29 30 31 32 33 34 35 36 37 38 39 40 41 42 43 44 45 46 47 48 49 50 51 52 53 54 55 56 57 58 59 60
| - (float *)detectInOpenCVWithSampleBuffer:(CMSampleBufferRef)sampleBuffer facePointCount:(int *)facePointCount isMirror:(BOOL)isMirror { cv::Mat cvImage = [self grayMatWithSampleBuffer:sampleBuffer]; int resultWidth = 250; int resultHeight = resultWidth * 1.0 / cvImage.rows * cvImage.cols; cvImage = [self resizeMat:cvImage toWidth:resultHeight]; cvImage = [self correctMat:cvImage isMirror:isMirror]; const char *imgData = (const char *)cvImage.data; int foundface; int len = 2 * stasm_NLANDMARKS; float *landmarks = (float *)malloc(len * sizeof(float)); int imgCols = cvImage.cols; int imgRows = cvImage.rows; const char *xmlPath = [[NSBundle mainBundle].bundlePath UTF8String]; int stasmActionError = stasm_search_single(&foundface, landmarks, imgData, imgCols, imgRows, "", xmlPath); if (!stasmActionError) { printf("Error in stasm_search_single: %s\n", stasm_lasterr()); } cvImage.release(); if (foundface) { for (int index = 0; index < len; ++index) { if (index % 2 == 0) { float scale = (self.videoSize.height / self.videoSize.width) / (16.0 / 9.0); scale = MAX(1, scale); landmarks[index] = (landmarks[index] / imgCols * 2 - 1) * scale; } else { float scale = (16.0 / 9.0) / (self.videoSize.height / self.videoSize.width); scale = MAX(1, scale); landmarks[index] = (landmarks[index] / imgRows * 2 - 1) * scale; } } *facePointCount = stasm_NLANDMARKS; return landmarks; } else { free(landmarks); return nil; } }
|
二、关键点绘制
通过上面的步骤,我们已经有了顶点数据,区别只是两种方式的顶点数量不同。
顶点数据的绘制,要在 GPUImageFilter
中进行。我们要自定义一个滤镜,然后在这个滤镜中实现人脸关键点的绘制逻辑。
在 GPUImageFilter
中,渲染的流程是在 -renderToTextureWithVertices:textureCoordinates:
这个方法里执行的。因此在自定义的滤镜中,我们需要重写这个方法。
在这个方法里,我们需要做两件事情,一是将输入的纹理原封不动地绘制,二是对人脸关键点的绘制。
纹理的绘制使用的是三角形图元,人脸关键点的绘制使用的是点图元,因此我们需要分成两次绘制。在原来的绘制方法中,已经有了纹理的绘制逻辑。所以,我们只需要在纹理绘制结束后,加上人脸关键点的绘制。
完整的重写后的方法:
1 2 3 4 5 6 7 8 9 10 11 12 13 14 15 16 17 18 19 20 21 22 23 24 25 26 27 28 29 30 31 32 33 34 35 36 37 38 39 40 41 42 43 44 45 46 47 48
| - (void)renderToTextureWithVertices:(const GLfloat *)vertices textureCoordinates:(const GLfloat *)textureCoordinates { if (self.preventRendering) { [firstInputFramebuffer unlock]; return; } [GPUImageContext setActiveShaderProgram:filterProgram]; outputFramebuffer = [[GPUImageContext sharedFramebufferCache] fetchFramebufferForSize:[self sizeOfFBO] textureOptions:self.outputTextureOptions onlyTexture:NO]; [outputFramebuffer activateFramebuffer]; if (usingNextFrameForImageCapture) { [outputFramebuffer lock]; } [self setUniformsForProgramAtIndex:0]; glClearColor(backgroundColorRed, backgroundColorGreen, backgroundColorBlue, backgroundColorAlpha); glClear(GL_COLOR_BUFFER_BIT); glActiveTexture(GL_TEXTURE2); glBindTexture(GL_TEXTURE_2D, [firstInputFramebuffer texture]); glUniform1i(filterInputTextureUniform, 2); glUniform1i(self.isPointUniform, 0); glVertexAttribPointer(filterPositionAttribute, 2, GL_FLOAT, 0, 0, vertices); glVertexAttribPointer(filterTextureCoordinateAttribute, 2, GL_FLOAT, 0, 0, textureCoordinates); glDrawArrays(GL_TRIANGLE_STRIP, 0, 4); if (self.facesPoints) { glUniform1i(self.isPointUniform, 1); glUniform1f(self.pointSizeUniform, self.sizeOfFBO.width * 0.006); glVertexAttribPointer(filterPositionAttribute, 2, GL_FLOAT, 0, 0, self.facesPoints); glDrawArrays(GL_POINTS, 0, self.facesPointCount); } [firstInputFramebuffer unlock]; if (usingNextFrameForImageCapture) { dispatch_semaphore_signal(imageCaptureSemaphore); } }
|
在绘制点图元的时候,可以通过对 gl_PointSize
进行赋值,来指定点的大小。然后在外部通过 uniform
变量传值的方式进行控制。
顶点着色器代码:
1 2 3 4 5 6 7 8 9 10 11 12 13 14 15
| precision highp float; attribute vec4 position; attribute vec4 inputTextureCoordinate; varying vec2 textureCoordinate; uniform float pointSize; void main() { gl_Position = position; gl_PointSize = pointSize; textureCoordinate = inputTextureCoordinate.xy; }
|
由于两次渲染的逻辑是独立的,所以一般来说,应该使用不同的 Shader 来实现。但由于这里的渲染逻辑比较简单,所以直接将两次渲染的逻辑都放到同一个 Shader 中。这也可以避免 Program 的来回切换,然后用一个 uniform
变量来判断当前的绘制类型。
片段着色器代码:
1 2 3 4 5 6 7 8 9 10 11 12 13 14 15 16
| precision highp float; varying vec2 textureCoordinate; uniform sampler2D inputImageTexture; uniform int isPoint; void main() { if (isPoint != 0) { gl_FragColor = vec4(1.0, 1.0, 1.0, 1.0); } else { gl_FragColor = texture2D(inputImageTexture, textureCoordinate); } }
|
最后,只需要将这个滤镜加入到滤镜链里,就可以看到人脸关键点的绘制效果了。
源码
请到 GitHub 上查看完整代码。
参考