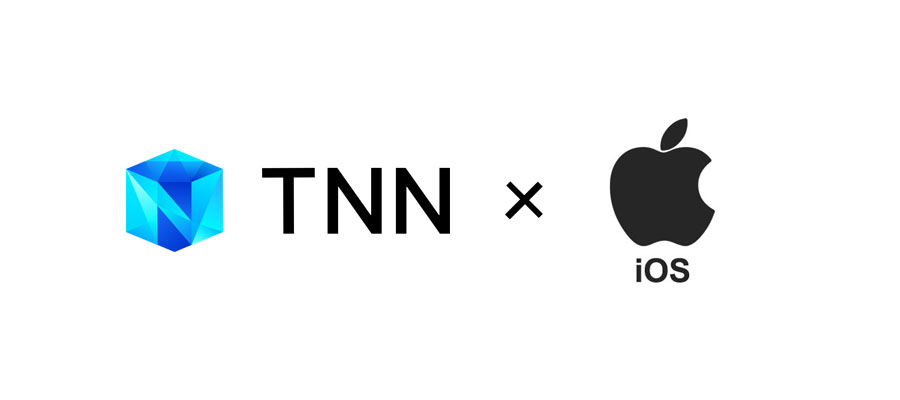
TNN 是腾讯优图实验室打造的高性能推理框架,目前已经在 GitHub 上开源,并在手Q、微视、P图等应用中落地。本文可能是比官方文档更加友好的入门指南,讲解如何从零在 iOS 工程中使用 TNN 框架。
TNN 是一个跨平台的框架,训练好的 TNN 模型可以直接在不同的平台使用。在官方介绍中没有 TNN 的全称,可能是 Tencent Neural Networks 。
目前,腾讯内部基于 TNN 框架落地的 AI 能力有 人脸识别、抠背、GAN 玩法 等。关于 TNN 的更多介绍可以到 GitHub 上查看。
下面以一个灰度图自动上色的例子,讲解 TNN 的使用流程。
〇、引入 SDK
在 新建的 iOS 工程 中引入 TNN SDK 分为三步:
- 编译 TNN 源码,生成
tnn.framework
和 tnn.bundle
,把它们加入工程,并添加工程配置。这一步可以按照官方的 TNN 编译指南 的 iOS 部分进行。
- 在
Build Phases->Link Binary With Libraries
中添加依赖库 Accelerate.framework
和 CoreML.framework
。
- 将默认生成的
ViewController.m
后缀改成 .mm
。
然后执行编译,没有报错则说明引入成功。
一、模型解析
模型包括两个文件,分别以 .tnnmodel
和 .tnnproto
为后缀。
解析的过程如下:
- 生成
TNN
实例。
- 读取
.tnnmodel
和 .tnnproto
的内容,封装成 ModelConfig
。
- 调用
TNN
的 Init
方法来加载 ModelConfig
。
关键代码:
1 2 3 4 5 6 7 8 9 10 11 12 13 14 15 16 17 18 19 20 21
| self.network = new TNN();
NSString *modelPath = [[NSBundle mainBundle] pathForResource:@"G_8_GRAY2RGB_256" ofType:@"tnnmodel"]; NSString *protoPath = [[NSBundle mainBundle] pathForResource:@"G_8_GRAY2RGB_256" ofType:@"tnnproto"];
string protoContent = [NSString stringWithContentsOfFile:protoPath encoding:NSUTF8StringEncoding error:nil].UTF8String; NSData *modelData = [NSData dataWithContentsOfFile:modelPath]; string modelContent = [modelData length] > 0 ? string((const char *)[modelData bytes], [modelData length]) : "";
ModelConfig modelConfig; modelConfig.model_type = MODEL_TYPE_TNN; modelConfig.params = {protoContent, modelContent};
Status status = self.network->Init(modelConfig);
|
二、构建网络
构建网络的步骤:
- 创建配置
NetworkConfig
,需要指定的参数有 device_type
和 library_path
。在移动端上一般用 GPU 来跑模型,所以在 iOS 上 device_type
一般用 DEVICE_METAL
。library_path
则指定 tnn.metallib
的路径。
- 调用
TNN
的 CreateInst
方法,加载 NetworkConfig
生成网络实例。
关键代码:
1 2 3 4 5 6 7 8 9 10
| NSString *bundlePath = [[NSBundle mainBundle] pathForResource:@"tnn" ofType:@"bundle"]; NSString *libPath = [bundlePath stringByAppendingPathComponent:@"tnn.metallib"];
Status status; NetworkConfig networkConfig; networkConfig.device_type = DEVICE_METAL; networkConfig.library_path = {libPath.UTF8String};
self.networkInstance = self.network->CreateInst(networkConfig, status);
|
三、预处理
每个模型都有特定的数据输入格式,大部分需要将图像 4 个通道的数据转化到 0 ~ 1
或者 -1 ~ 1
范围。所以在执行网络之前,需要一个预处理步骤。
在 TNN 中,模型可以理解成封装好的「函数」,我们只需要关心「函数」的输入输出,「函数」的具体实现可以不用管。
TNN 中模型的输入输出是 Blob
格式,模型可以支持多个 Blob
的输入和多个 Blob
的输出。这个例子的模型是单输入单输出。
在进行预处理之前,先要获取输入的 Blob
:
1 2 3
| BlobMap inputBlobs; Status status = self.networkInstance->GetAllInputBlobs(inputBlobs); Blob *networkInput = inputBlobs.begin()->second;
|
另外,还需要获取准备输入的图像数据,这里是一个 Metal 的纹理:
1 2 3 4 5
| MTKTextureLoader *textureLoader = [[MTKTextureLoader alloc] initWithDevice:MTLCreateSystemDefaultDevice()]; NSDictionary *options = @{ MTKTextureLoaderOptionSRGB : @NO }; id<MTLTexture> texture = [textureLoader newTextureWithCGImage:image.CGImage options:options error:NULL];
|
然后,可以使用两种方式进行预处理:默认方式、自定义方式 。
1、默认方式
前面说到,预处理的目的大部分是进行数值范围的转化,所以 TNN 提供了一种很方便的转化方式,可以通过 scale
、bias
参数来设置转化的范围,还可以通过 reverse_channel
来设置通道转换,例如将 RGB
转成 BGR
。
参考代码:
1 2 3 4 5 6 7 8 9
| Mat inputMat = {DEVICE_METAL, tnn::N8UC4, (__bridge void*)texture}; shared_ptr<BlobConverter> preprocessor = make_shared<BlobConverter>(networkInput);
id<MTLCommandQueue> commandQueue = [self fetchCommandQueue]; MatConvertParam input_cvt_param; input_cvt_param.scale = {2.0 / 255, 2.0 / 255, 2.0 / 255, 0}; input_cvt_param.bias = {-1.0, -1.0, -1.0, 0}; input_cvt_param.reverse_channel = true; preprocessor->ConvertFromMatAsync(inputMat, input_cvt_param, (__bridge void*)commandQueue);
|
上面的转化参数可以将 0 ~ 255
的范围转到 -1 ~ 1
。
设置好转化的参数后,调用 ConvertFromMatAsync
执行预处理。
2、自定义方式
如果模型的输入格式比较特异化,只通过 scale
、bias
无法进行调整,则需要使用自定义的方式。
自定义的方式需要先获取输入 Blob
的 MTLBuffer
,然后构造 MTLComputePipelineState
,实现自定义的 kernel
函数,将预处理的结果写到 MTLBuffer
中。
参考代码:
1 2 3 4 5 6 7 8 9 10 11 12 13 14 15 16 17 18 19 20 21 22 23
| id<MTLCommandQueue> commandQueue = [self fetchCommandQueue]; id<MTLCommandBuffer> commandBuffer = [commandQueue commandBuffer]; [commandBuffer enqueue];
id<MTLBuffer> blobBuffer = (__bridge id<MTLBuffer>)(void *)networkInput->GetHandle().base; NSUInteger blobOffset = (NSUInteger)networkInput->GetHandle().bytes_offset;
id<MTLComputeCommandEncoder> encoder = [commandBuffer computeCommandEncoder];
id<MTLComputePipelineState> pipelineState = [self computePipelineStateWithLibrary:self.library functionName:@"test_preprocess"]; [encoder setComputePipelineState:pipelineState]; [encoder setTexture:texture atIndex:0]; [encoder setBuffer:blobBuffer offset:blobOffset atIndex:0];
NSUInteger width = pipelineState.threadExecutionWidth; NSUInteger height = pipelineState.maxTotalThreadsPerThreadgroup / width; MTLSize groupThreads = {width, height, (NSUInteger)1}; MTLSize groups = {((texture.width + width - 1) / width), ((texture.height + height - 1) / height), 1}; [encoder dispatchThreadgroups:groups threadsPerThreadgroup:groupThreads]; [encoder endEncoding];
[commandBuffer commit]; [commandBuffer waitUntilScheduled];
|
这里的 dispatchThreadgroups:threadsPerThreadgroup:
是在设置 GPU 并发处理时的线程组划分和每个线程组的线程数。这是官方比较推荐的写法,详情可以查看 官方文档 。
预处理的 kernel 函数:
1 2 3 4 5 6 7 8 9 10 11 12 13 14 15 16 17
| kernel void test_preprocess(texture2d<half, access::read> inputTexture [[texture(0)]], device half4 *dst [[buffer(0)]], ushort2 gid [[thread_position_in_grid]]) { int width = inputTexture.get_width(); int height = inputTexture.get_height(); if (any(gid >= ushort2(width, height))) { return; }
const half4 in = inputTexture.read(uint2(gid)); auto out = dst + (int)gid.y * width + (int)gid.x; *out = half4(in.x * 2 - 1, in.y * 2 - 1, in.z * 2 - 1, 0.0); }
|
这里做的事情是从 inputTexture
中读取数据,然后写到 dst
中。
四、执行网络
预处理之后,调用 ForwardAsync
来执行网络。
1
| self.networkInstance->ForwardAsync([]{});
|
五、后处理
与预处理类似,网络执行之后输出的数据,一般不能直接拿来使用。比如大部分模型输出的数据是 0 ~ 1
或者 -1 ~ 1
范围,而图像 4 个通道的数据是 0 ~ 255
,所以还需要一个后处理步骤。
后处理与预处理一样,也有 默认 和 自定义 两种方式。只不过后处理取的是输出的 Blob
。
1、默认方式
预处理是调用 ConvertFromMatAsync
来执行,而后处理是调用 ConvertToMatAsync
来执行。
参考代码:
1 2 3 4 5 6 7 8 9
| Mat outputMat = {DEVICE_METAL, tnn::N8UC4, (__bridge void*)texture}; shared_ptr<BlobConverter> postprocessor = make_shared<BlobConverter>(networkOutput);
id<MTLCommandQueue> commandQueue = [self fetchCommandQueue]; MatConvertParam output_cvt_param; output_cvt_param.scale = {255 / 2.0, 255 / 2.0, 255 / 2.0, 0}; output_cvt_param.bias = {255 / 2.0, 255 / 2.0, 255 / 2.0, 255}; output_cvt_param.reverse_channel = true; postprocessor->ConvertToMatAsync(outputMat, output_cvt_param, (__bridge void*)commandQueue);
|
2、自定义方式
使用自定义方式时,后处理的代码与预处理基本相同,只是加载的 kernel 函数不同。
参考代码:
1 2 3 4 5 6 7 8 9 10 11 12 13 14 15 16 17 18 19 20 21 22 23
| id<MTLCommandQueue> commandQueue = [self fetchCommandQueue]; id<MTLCommandBuffer> commandBuffer = [commandQueue commandBuffer]; [commandBuffer enqueue];
id<MTLBuffer> blobBuffer = (__bridge id<MTLBuffer>)(void *)networkOutput->GetHandle().base; NSUInteger blobOffset = (NSUInteger)networkOutput->GetHandle().bytes_offset; id<MTLComputeCommandEncoder> encoder = [commandBuffer computeCommandEncoder];
id<MTLComputePipelineState> pipelineState = [self computePipelineStateWithLibrary:self.library functionName:@"test_postprocess"]; [encoder setComputePipelineState:pipelineState]; [encoder setTexture:texture atIndex:0]; [encoder setBuffer:blobBuffer offset:blobOffset atIndex:0];
NSUInteger width = pipelineState.threadExecutionWidth; NSUInteger height = pipelineState.maxTotalThreadsPerThreadgroup / width; MTLSize groupThreads = {width, height, (NSUInteger)1}; MTLSize groups = {((texture.width + width - 1) / width), ((texture.height + height - 1) / height), 1}; [encoder dispatchThreadgroups:groups threadsPerThreadgroup:groupThreads]; [encoder endEncoding];
[commandBuffer commit]; [commandBuffer waitUntilScheduled];
|
对应的 kernel 函数实现如下:
1 2 3 4 5 6 7 8 9 10 11 12 13 14 15 16
| kernel void test_postprocess(texture2d<half, access::write> outputTexture [[texture(0)]], const device half4 *src0 [[buffer(0)]], uint2 gid [[thread_position_in_grid]]) { int width = outputTexture.get_width(); int height = outputTexture.get_height(); if (any(gid >= uint2(width, height))) { return; } half4 in = src0[(int)gid.y * width + (int)gid.x]; in.x = (in.x + 1) / 2; in.y = (in.y + 1) / 2; in.z = (in.z + 1) / 2; in.w = 1; outputTexture.write(in, uint2(gid)); }
|
这里做的事情是从 src0
中读取数据,然后写到 outputTexture
中。
最后看一下效果:
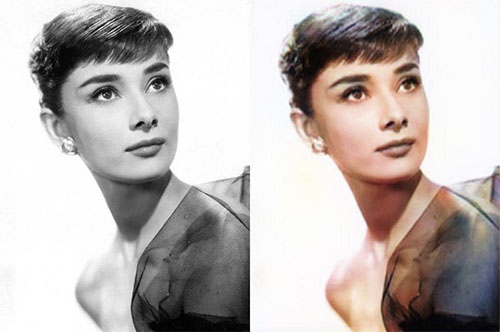
源码
请到 GitHub 上查看完整代码。